AI as Ungoverned Protozoan Life
When it encounters reality, its biases and limitations can do real harm
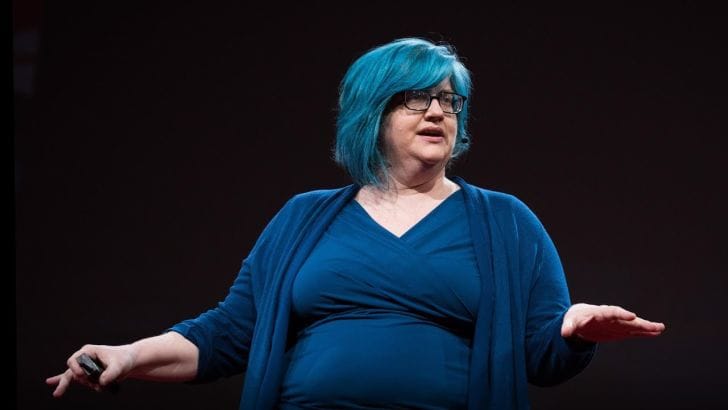
One of the best phrases I’ve seen recently is, “over-described and under-conceptualized.” We hear artificial intelligence (AI) described again and again, but do we really understand the concepts it should and hopefully will ultimately encompass? Is it a technology question? Or are the questions much more fundamental — about rights, obligations, freedom, equality, and justice?
Over decades and centuries, the legal system developed elements that AI currently does not possess — an appeals process, the writ of habeas corpus, enumeration of rights, common law standards, admissibility criteria for evidence/data, and the presumption of innocence. Civil and criminal statutes were created, challenged, overturned, and revised.
The seriousness with which we need to approach AI seems especially salient given how Google disbanded its AI ethics panel within one week of its announcement, after Google employees petitioned against the inclusion of a person they described as “vocally anti-trans, anti-LGBTQ, and anti-immigrant.” Google defended this individual’s inclusion on the basis of “diversity of thought,” confirming their inability to differentiate between legitimate and illegitimate diversity of opinion — probably another reason they can’t control hate speech, as well. Others on the panel resigned, and things fell apart quickly. For Google, the AI ethics panel was a veneer to apply to its technology, a heat shield, but nothing they took very seriously. Disbanding it probably caused few at Google to lose sleep.
Yet, AI is affecting lives every day while none of these issues have been fleshed out. Data transparency (the ability to review the “evidence”)? Appeals process? The presumption of innocence? Privacy at the micro and macro levels? Enumeration of rights?
So it’s no surprise given the current immature state of the AI conceptualization process, smart researchers — many from under-represented groups in tech — have revealed a variety of problems with the current state of AI, from bias to injustice to intrusion to inaccuracy to harm.
Rudimentary AI is showing up in the strangest places. Back in 2016, I reviewed Cathy O’Neil’s book, “Weapons of Math Destruction,” in which she covered some of these strange places AI is making an appearance, including shift-scheduling software for part-time workers — a development that is leading to stranger schedules and less predictable hours (and therefore wages) for hourly workers, with O’Neil writing:
It’s almost as if the software were designed expressly to punish low-wage workers and to keep them down.
You can see an informative TED Talk from O’Neil here:
She opens with the fact that we are sorted, ranked, and ordered without the ability to appeal these machine-learning decisions. Again, we have no rights. A separate AI society in which we are merely fodder for machine learning techniques is being created, with a few humans controlling it.
O’Neil is not the only one raising concerns. In 2017, the AI Now Institute was founded with the goal of producing interdisciplinary research on the social implications of artificial intelligence, focusing on rights and liberties, labor and automation, bias and inclusion, and safety and critical infrastructure. It is currently housed at NYU. The AI Now Institute was founded by Kate Crawford and Meredith Whittaker, who were recently interviewed on the “Recode Decode” podcast about AI and issues they’ve identified with it during their research and careers.
One of the biggest issues is how AI currently indulges in stereotyping, with Whittaker noting that even simple AI-assisted tasks like “search” are biased in unacceptable ways. She tells the story of running a search on “CEO,” and finding a huge list of what she calls “white dudes”:
. . . the first female CEO that came up in these searches that we were running at the time was Barbie CEO, and you’re like, “Okay, that’s a problem.” And it’s funny because it’s like a whack-a-mole problem right now. Right? So industry is like, “Oh, okay, we see a problem.” That we’re actually, if you look up “physicist” right now, you’ll still see some differences. So again, around professions around these cliche stereotypes around gender and race, you keep seeing them get reflected and try to fix it.
So, we have two big problems revealed here — AI that is biased in obvious ways that any careful observer of the world would see; and the fact that such AI has been deployed on a broad scale (Google), and now has to be fixed ex post facto even as it affects how people see the world and how the world sees them.
AI has been over-described and under-conceptualized.
One major challenge with any data-driven system is what is commonly called “garbage in, garbage out,” which refers to bad data being used in systems that transform the bad data into unreliable or inaccurate outputs. This is GIGO. Whittaker calls it “crap in, even weirder crap out,” which would abbreviate as CIEWCO, which I like to pronounce as “sewko.” You could also spell it as “SIEWSO,” or “sewso.”
The challenges go on from there, and ultimately — like most technology issues — involve people. Part of the reason Barbie CEO is the first female CEO in Google stems from the lack of diversity in the workforce. This was not always the case. The computer science and AI fields have become less diverse over time, with lower and lower percentages of females, non-whites, and non-engineers involved. As Crawford notes, it wasn’t always this way. We used to be much more thoughtful:
. . . if you go back in the history of AI, to the beginning, in the 1950s and ’60s, it was a much more diverse field. You had anthropologists sitting at the table with computer scientists. It was this vision of how do we construct a world that we want to live in. . . . right now, as we have these real issues of homogeneity in Silicon Valley. We need to open those doors up, but we also need to get people in the room who are the ones who are most likely to be seeing the downsides of the system. We have to center affected communities and not just engineers on big salaries.
How did technology become so dominated by a homogenous “bro culture”? As Sara Wachter-Boettcher wrote in her book, “Technically Wrong,” this trend may have been exacerbated by the emergence of the PC:
Originally, programming was often categorized as “women’s work,” lumped in with administrative skills like typing and dictation (in fact, during World War II, the word “computers” was often applied not to machines, but to the women who used them to compute data). As more colleges started offering computer science degrees, in the 1960s, women flocked to the programs: 11 percent of computer science majors in 1967 were women. By 1984, that number had grown to 37 percent. Starting in 1985, that percentage fell every single year — until, in 2007, it leveled out at the 18 percent figure we saw through 2014. That shift coincides perfectly with the rise of the personal computer.
Crawford, from the AI Now Institute, relays a story about biased HR systems that use AI to scan résumés:
In many cases, decisions are gonna be made about you. You’re not even aware that an AI system is working in the background. Let’s take HR for a classic case in point right now. Now, many of you have probably tried sending CVs and résumés in to get a job. What you may not know is that in many cases, companies are using AI systems to scan those résumés, to decide whether or not you’re worthy of an interview, and that’s fine until you start hearing about Amazon’s system, where they took two years to design, essentially, an AI automatic résumé scanner.
And they found that it was so biased against any female applicant that if you even had the word “woman” on your résumé that it went to the bottom of the pile. I mean, it was extraordinary.
Crawford and Whittaker are not impressed by the “intelligence” of AI, comparing it at one point to intelligence at the “protozoan level.” Yet, we allow this dim intelligence to infiltrate all manner of decisions, and defer to it all too often. We also have few if any methods of remediation or appeal if the AI does something wrong.
The interview is fascinating and informative, and I recommend reading or listening to it all. These two leaders in AI research do see hope with lawmakers, with Crawford reflecting on how regulatory bodies are evolving:
I’ll say that there are some really interesting senators, right now, who are asking different questions. They’re looking at algorithmic accountability. That’s really key to see. They’re having different conversations about privacy that realize that it’s not just about individual privacy, it’s about our collective privacy. It’s the fact that, if you make a decision in a social media network, that can affect how data from all of your contacts is being extracted, as well. I think there’s an increasing level of literacy, and that’s something that’s super important.
However, governance on a patchwork basis isn’t probably the solution, and Crawford notes later that when a similarly powerful technology — nuclear weapons and nuclear power — was created last century, there was a worldwide effort to create a single governing system. She’s not seeing something like that happening now, as the political moment is far different, and also the appreciation of the power of AI is too variable. Images of Nagasaki or Hiroshima, or films of nuclear detonations, galvanized opinion of the dangers of nuclear weapons globally. The power of AI is far more subtle, even unknown or unknowable, so people are less motivated to rein it in. Perhaps the decimation of the US and the UK political systems by AI platforms exploited by adversaries may have woken some people up.
It’s encouraging to see what the AI Now Institute is doing — thinking and researching. We need more of that, and less wanton implementation of unprecedented technologies we barely comprehend but which we allow to scale quickly, impose unknown biases, change lives, and break things.
Let’s over-conceptualize for once. Let’s think this through with actual intelligence.